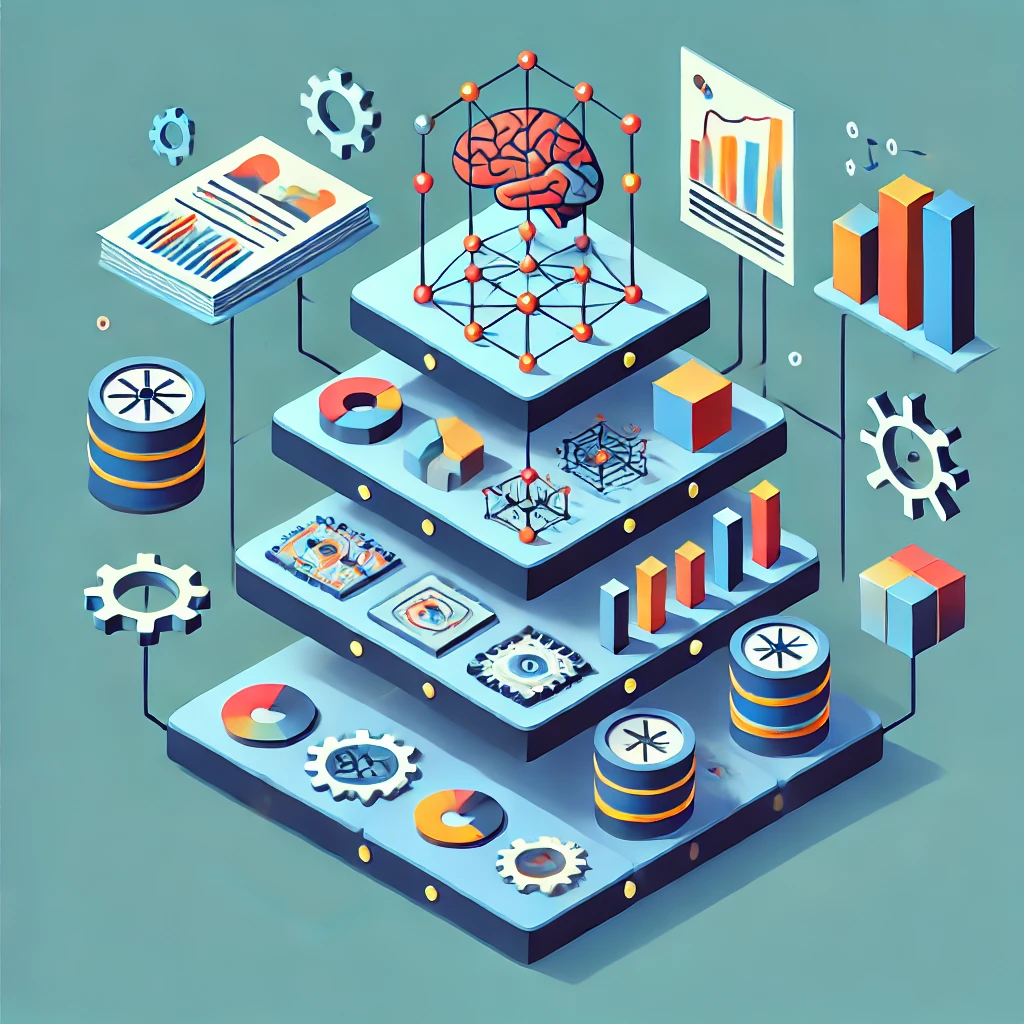
Machine learning is a branch of artificial intelligence that permits systems to study from facts and improve performance without specific programming. Machine mastering algorithms can optimize approaches and beautify efficiency in various industries by studying patterns and making fact-based choices.
Efficiency and productivity are key drivers of success in manufacturing environments. By leveraging device learning technology, agencies can streamline operations, reduce costs, and enhance the quality of their merchandise, ultimately gaining a competitive edge in the market.
Applications of Machine Learning in Production
Machine Learning finds extensive application in optimizing production schedules and resource allocation, particularly in applications of machine learning in manufacturing, revolutionizing efficiency and quality across industries. By leveraging predictive analytics, ML algorithms decrease downtime and maximize output. Additionally, machine learning fashions enhance product first-class via real-time anomaly detection and predictive renovation, ensuring steady overall performance and reducing costly defects.
Quality Control: Machine learning algorithms can analyze large volumes of data to detect defects and anomalies in real-time, improving quality control processes and reducing the risk of defective products reaching customers.
Predictive Maintenance: By analyzing historical data and sensor readings, machine learning models can predict when equipment will likely fail, enabling proactive maintenance and reducing downtime.
Demand Forecasting: Machine learning algorithms can analyze past sales data, market trends, and other variables to forecast future demand accurately, helping companies optimize inventory levels and production schedules.
Process Optimization: Machine learning can optimize production processes by identifying bottlenecks, minimizing waste, and improving efficiency.
Benefits of Using Machine Learning in Production
Using Machine Learning in production offers numerous benefits. It optimizes operations by predicting renovation desires and reducing downtime, enhancing overall performance. ML improves product best via real-time anomaly detection and system optimization, ensuring constant output and minimizing defects. Moreover, it allows facts-pushed selection-making, leading to better resource allocation and price savings across the manufacturing lifecycle.
Cost Reduction: Machine learning can help companies minimize costs and maximize profitability by increasing efficiency, reducing downtime, and optimizing processes.
Increased Efficiency: Machine learning algorithms can automate repetitive tasks, optimize workflows, and make real-time adjustments, increasing productivity and throughput.
Enhanced Product Quality: By improving quality control processes and detecting defects early, machine learning can help ensure that products meet the highest quality standards.
Real-time Decision Making: Machine learning enables real-time data analysis, allowing companies to make informed decisions quickly and adapt to changing conditions in the production environment.
Challenges and Considerations
Implementing Machine Learning in production presents several challenges and considerations. These encompass the want for robust records infrastructure to assist large-scale data processing and model education. Additionally, ensuring model interpretability and reliability is crucial for gaining trust in automated decision-making processes. Furthermore, addressing scalability issues and integrating ML systems seamlessly into existing production workflows requires careful planning and expertise. Overcoming these challenges involves a multidisciplinary approach, combining domain knowledge with advanced analytics and engineering skills to achieve sustainable deployment and optimization of ML solutions in production environments.
Data Quality and Availability: Machine learning models rely on high-quality data for training and decision-making. Ensuring data accuracy and availability can be a challenge in production environments.
Integration with Existing Systems: Integrating machine learning solutions with existing production systems and workflows can be complex and require careful planning to ensure seamless operation.
Maintenance and Upkeep of ML Models: Machine learning models require regular maintenance and updates to remain effective. Companies need to allocate resources for ongoing model training and optimization.
Case Studies and Examples
Manufacturing industry
Case Study 1: Predictive Maintenance
In manufacturing, downtime due to unplanned equipment failure can be costly. By implementing machine learning for predictive maintenance, companies can proactively identify equipment likely to fail and schedule maintenance activities accordingly. For example, a leading automotive manufacturer used machine learning algorithms to analyze sensor data from their assembly line machinery. By detecting patterns indicative of potential failures, they were able to schedule maintenance activities during planned downtime, reducing unplanned downtime by 20% and saving millions of dollars annually.
Case Study 2: Quality Control
Ensuring product quality is paramount in manufacturing. Machine learning algorithms can analyze data from various sensors and cameras to identify defects and anomalies in real time, allowing manufacturers to take immediate corrective actions. An electronics manufacturer implemented a machine learning system that analyzed images of electronic components during production. This enabled them to detect even the smallest defects that would have gone unnoticed. As a result, they significantly reduced the number of defective products reaching customers and improved overall product quality.
Energy sector
Case Study 1: Demand Forecasting
Accurate demand forecasting is crucial to optimize energy generation and distribution in the energy sector. Machine learning algorithms can analyze historical energy consumption data, weather patterns, and other variables to forecast future demand accurately. A utility company implemented a machine learning solution to forecast electricity demand on a regional level. By leveraging this technology, they could adjust power generation and distribution in real-time, ensuring optimal utilization of resources and avoiding potential strain on the electricity grid.
Case Study 2: Asset Management
Proper management and maintenance of energy assets are vital for efficient operations. Machine learning can help identify maintenance needs and optimize asset management strategies. For instance, a renewable energy company used machine learning to analyze data from wind turbines. By detecting performance degradation and predicting maintenance needs in advance, they could reduce downtime and optimize maintenance schedules, resulting in increased productivity and improved asset lifespan.
Retail and supply chain
Case Study 1: Inventory Management
Efficient inventory management is critical in retail and supply chain operations. By leveraging machine learning, companies can analyze historical sales data, seasonality patterns, and market trends to optimize inventory levels and reduce carrying costs. A supermarket chain implemented machine learning algorithms to forecast demand for various product categories. By aligning inventory levels with demand, they achieved significant cost savings by reducing excess inventory and stockouts.
Case Study 2: Personalized Recommendations
Machine learning is also used to provide personalized recommendations to customers, enhancing their shopping experience. A leading e-commerce company utilizes machine learning algorithms to analyze customer browsing and purchasing history. By leveraging these statistics, they may offer personalized product guidelines, increasing client engagement, higher conversion prices, and improved customer satisfaction.
These case studies demonstrate how machine learning transforms various industries, from manufacturing to energy and retail. By harnessing the power of data and algorithms, companies can optimize their operations, improve efficiency, and deliver higher-quality products and services.
Conclusion
Machine learning offers significant benefits for improving production processes, including cost reduction, increased efficiency, enhanced product quality, and real-time decision-making.
As machine learning technologies advance, the potential for improving production processes will only grow. Companies that embrace machine learning solutions stand to gain a competitive advantage and drive innovation in their industries.
In conclusion, machine learning in production holds immense potential for driving efficiency, reducing costs, and enhancing product quality. By harnessing the power of data and algorithms, businesses can optimize their operations and gain a competitive edge in today’s fast-paced markets.